Adversarial Fairness
Quick Navigation:
- Adversarial Fairness Definition
- Adversarial Fairness Explained Easy
- Adversarial Fairness Origin
- Adversarial Fairness Etymology
- Adversarial Fairness Usage Trends
- Adversarial Fairness Usage
- Adversarial Fairness Examples in Context
- Adversarial Fairness FAQ
- Adversarial Fairness Related Words
Adversarial Fairness Definition
Adversarial Fairness is an approach within AI and machine learning that seeks to mitigate biases by using adversarial models, which are frameworks designed to actively challenge and correct unfair outcomes. In adversarial fairness, two networks—typically, a predictor and a discriminator—work in opposition: the predictor aims to make unbiased predictions, while the discriminator detects and challenges biases. This dynamic fosters a fairer, more balanced AI model, especially valuable in fields like hiring algorithms, lending, and healthcare predictions.
Adversarial Fairness Explained Easy
Imagine a game where two players have different goals. One tries to guess your favorite color without making any wrong guesses, and the other checks if they're being fair by making sure all colors get equal attention. Adversarial Fairness is like this, with one model making decisions and another checking if those decisions are fair to everyone.
Adversarial Fairness Origin
The concept of Adversarial Fairness emerged from the need to address biases in machine learning, where models often exhibit prejudices due to biased data or inherent flaws in training algorithms. Researchers expanded adversarial training techniques, primarily used in Generative Adversarial Networks (GANs), to challenge and adjust unfair decisions in AI.
Adversarial Fairness Etymology
The term stems from "adversarial," referring to the confrontational role in training, paired with "fairness" to emphasize the aim of achieving impartial outcomes.
Adversarial Fairness Usage Trends
Interest in Adversarial Fairness has grown rapidly, as societal awareness of AI bias has increased. Its usage spans industries, especially in applications like HR tech, financial services, and automated healthcare, where fair treatment across different groups is essential. Governments and tech companies alike are implementing this approach to promote ethical standards in AI systems.
Adversarial Fairness Usage
- Formal/Technical Tagging:
- Machine Learning
- Bias Mitigation
- Fairness Algorithms - Typical Collocations:
- "adversarial fairness in AI"
- "fairness-driven adversarial model"
- "bias detection with adversarial techniques"
Adversarial Fairness Examples in Context
- An adversarial fairness algorithm helps ensure that a loan approval model is fair by challenging it to make unbiased predictions across different income groups.
- In hiring software, adversarial fairness techniques are used to prevent bias against certain genders by training models to ignore irrelevant demographic features.
- Adversarial fairness in healthcare AI promotes unbiased patient diagnoses by pushing models to consider symptoms fairly across ethnic groups.
Adversarial Fairness FAQ
- What is Adversarial Fairness?
Adversarial Fairness is a machine learning technique designed to minimize biases by employing adversarial models. - How does Adversarial Fairness work?
It works by having two networks: one to make predictions and another to detect biases, ensuring the outcomes are fair. - Why is Adversarial Fairness important?
It helps prevent unfair decisions by addressing biases in AI models, especially in sensitive applications. - Can Adversarial Fairness apply to hiring processes?
Yes, it is used to ensure unbiased hiring by training models to ignore irrelevant demographic factors. - Is Adversarial Fairness complex to implement?
It can be challenging, as it requires designing multiple networks that interact effectively to reduce biases. - Which industries use Adversarial Fairness?
Adversarial fairness is widely used in finance, HR, healthcare, and any field concerned with fair AI predictions. - How does Adversarial Fairness relate to GANs?
It builds on GANs by applying adversarial principles to bias detection rather than image generation. - Can Adversarial Fairness guarantee unbiased AI?
While it reduces biases, it cannot guarantee a completely unbiased model due to complexities in data and model design. - What are some challenges in Adversarial Fairness?
Key challenges include data limitations, complexity in model design, and computational costs. - What is the future of Adversarial Fairness?
With ongoing AI ethics research, adversarial fairness is likely to evolve, becoming more efficient and widely applicable.
Adversarial Fairness Related Words
- Categories/Topics:
- Ethical AI
- Bias Detection
- Machine Learning Fairness
Did you know?
Adversarial Fairness techniques were first explored in high-stakes environments like criminal justice, where biased AI decisions had significant consequences. By employing adversarial fairness, models could better ensure equitable treatment across demographic lines, leading to more just AI applications.
Authors | Arjun Vishnu | @ArjunAndVishnu
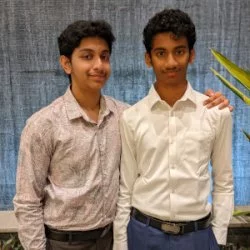
PicDictionary.com is an online dictionary in pictures. If you have questions or suggestions, please reach out to us on WhatsApp or Twitter.
I am Vishnu. I like AI, Linux, Single Board Computers, and Cloud Computing. I create the web & video content, and I also write for popular websites.
My younger brother, Arjun handles image & video editing. Together, we run a YouTube Channel that's focused on reviewing gadgets and explaining technology.
Comments (0)
Comments powered by CComment