Algorithmic Accountability
Quick Navigation:
- Algorithmic Accountability Definition
- Algorithmic Accountability Explained Easy
- Algorithmic Accountability Origin
- Algorithmic Accountability Etymology
- Algorithmic Accountability Usage Trends
- Algorithmic Accountability Usage
- Algorithmic Accountability Examples in Context
- Algorithmic Accountability FAQ
- Algorithmic Accountability Related Words
Algorithmic Accountability Definition
Algorithmic accountability refers to the practices and policies that hold developers and deployers of algorithms responsible for the outcomes of their creations. It aims to ensure that algorithms, especially in sensitive areas like hiring, policing, and finance, are fair, transparent, and unbiased. By enforcing standards of accountability, stakeholders can mitigate risks associated with algorithmic decisions and foster trust in technology. This concept involves auditing algorithms to detect biases, ensuring transparency in their operations, and establishing regulatory frameworks to prevent misuse.
Algorithmic Accountability Explained Easy
Imagine a teacher using a scoring sheet to grade students but not explaining the criteria. If students feel that their grades aren't fair, they’d want an explanation of how the scores are assigned. Algorithmic accountability is similar—it makes sure algorithms are fair and transparent so people understand the rules they follow.
Algorithmic Accountability Origin
Algorithmic accountability emerged with the increasing integration of algorithms in decision-making processes, especially where these decisions impact people directly. As AI technologies became prevalent in the early 21st century, society recognized the need for safeguards against unintended biases or discrimination embedded within automated systems. Academic research, government regulations, and industry standards are contributing to shaping accountability frameworks.
Algorithmic Accountability Etymology
The term "algorithmic accountability" combines “algorithm,” a structured set of instructions, and “accountability,” meaning responsibility for outcomes.
Algorithmic Accountability Usage Trends
Interest in algorithmic accountability has risen with advances in AI and big data analytics. Public concern over AI-driven decisions, especially in legal and financial contexts, has pushed for stricter accountability measures. In response, regulatory frameworks are evolving worldwide, mandating that companies disclose how algorithms make decisions, with some requiring periodic audits to ensure transparency.
Algorithmic Accountability Usage
- Formal/Technical Tagging:
- Artificial Intelligence
- Ethics in Technology
- Machine Learning Compliance - Typical Collocations:
- "algorithmic accountability framework"
- "ethical AI"
- "algorithm bias auditing"
- "accountability in machine learning"
Algorithmic Accountability Examples in Context
- Tech companies are adopting algorithmic accountability frameworks to address potential biases in hiring algorithms.
- Government agencies use algorithmic accountability to regulate AI applications, ensuring they don’t perpetuate discrimination.
- Some banks apply algorithmic accountability measures to their lending algorithms to avoid biased credit assessments.
Algorithmic Accountability FAQ
- What is algorithmic accountability?
Algorithmic accountability ensures that algorithms operate in a transparent, fair, and responsible manner. - Why is algorithmic accountability important?
It protects individuals and communities from potential harms caused by biased or opaque algorithms. - How is algorithmic accountability achieved?
Through regular auditing, transparency measures, and adherence to ethical standards. - Which industries use algorithmic accountability?
Sectors like finance, healthcare, criminal justice, and hiring have accountability measures in place for AI. - What are some challenges in implementing algorithmic accountability?
Issues include lack of transparency, technical complexity, and difficulty in detecting biases. - Can algorithmic accountability reduce biases?
Yes, it identifies and corrects biases, promoting fairness in AI-driven decisions. - Is there a legal requirement for algorithmic accountability?
In some regions, laws mandate companies to explain and audit algorithmic decisions. - How do companies audit algorithms?
They review data inputs, testing results, and bias detection mechanisms to assess fairness. - What role do ethics play in algorithmic accountability?
Ethics guide the principles of fairness and transparency in algorithms. - Does algorithmic accountability affect AI development?
Yes, developers integrate accountability features to meet ethical and legal standards.
Algorithmic Accountability Related Words
- Categories/Topics:
- Ethics in Technology
- Bias Detection
- Data Responsibility
Did you know?
In 2020, a major social media platform implemented algorithmic accountability policies after its algorithms were shown to amplify certain content unfairly. This change sparked industry-wide discussions on ethical AI and transparency.
Authors | @ArjunAndVishnu
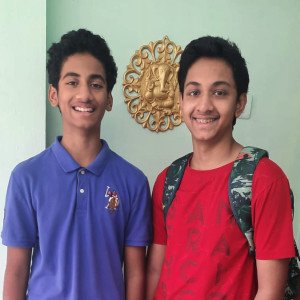
PicDictionary.com is an online dictionary in pictures. If you have questions, please reach out to us on WhatsApp or Twitter.
I am Vishnu. I like AI, Linux, Single Board Computers, and Cloud Computing. I create the web & video content, and I also write for popular websites.
My younger brother Arjun handles image & video editing. Together, we run a YouTube Channel that's focused on reviewing gadgets and explaining technology.
Comments powered by CComment