Data Labeling
Quick Navigation:
- Data Labeling Definition
- Data Labeling Explained Easy
- Data Labeling Origin
- Data Labeling Etymology
- Data Labeling Usage Trends
- Data Labeling Usage
- Data Labeling Examples in Context
- Data Labeling FAQ
- Data Labeling Related Words
- Did you know?
Data Labeling Definition
Data labeling is the process of tagging or annotating data—typically images, text, or videos—to make it understandable and usable by machine learning models. In supervised learning, labeled data provides the “ground truth” needed to train models. This process involves identifying and categorizing elements within a dataset to give the machine learning algorithm a reliable basis for recognizing patterns and making predictions. Data labeling can include tasks like marking objects in images, categorizing text, or transcribing audio, and it is essential for the accuracy and performance of AI models, especially in tasks like image recognition, natural language processing, and speech recognition.
Data Labeling Explained Easy
Imagine you’re looking at a picture of animals, and your job is to put a label on each one, like “cat,” “dog,” or “rabbit.” Data labeling is like that but for computers! By telling a computer what each thing is, you’re helping it learn so that it can figure things out on its own the next time it sees a similar picture. This makes it easier for AI to recognize things like animals, words, or even voices.
Data Labeling Origin
Data labeling became popular with the rise of supervised machine learning in the 1980s and 1990s when researchers started realizing that labeled data was necessary to train AI models. With the boom in AI applications over the past decade, data labeling has grown into a large-scale industry, driven by demand from sectors like technology, healthcare, finance, and more.
Data Labeling Etymology
The term "labeling" comes from the Latin word labelium, meaning “small piece of paper for a tag or sign.” This concept evolved into marking or annotating in the context of data, where each label gives identifiable information to help computers understand patterns.
Data Labeling Usage Trends
Data labeling has seen exponential growth, particularly with the expansion of AI-driven technologies in various sectors. The rise of machine learning, especially deep learning, has driven a demand for high-quality labeled datasets. Today, industries such as retail, healthcare, and autonomous vehicles heavily rely on labeled data. Companies now outsource data labeling to third-party firms, while some leverage crowdsourcing platforms to handle large volumes.
Data Labeling Usage
- Formal/Technical Tagging: Image annotation, text categorization, segmentation, tagging
- Typical Collocations: Data annotation, labeled data, ground truth, supervised learning, machine learning dataset
Data Labeling Examples in Context
"To improve the accuracy of its AI model, the tech company invested in extensive data labeling for millions of images."
"Data labeling companies have emerged as key players in the AI industry, providing services to train machine learning models."
"For our project, we need to manually label data to create a reliable training dataset for the language model."
Data Labeling FAQ
- What is data labeling in AI?
Data labeling is the process of tagging data with relevant information so AI models can recognize patterns. - Why is data labeling important?
It provides the necessary training data that enables machine learning models to make accurate predictions. - How is data labeling done?
Data can be labeled manually by human annotators or automatically using AI-based tools. - Who uses data labeling services?
Industries like technology, healthcare, retail, and autonomous vehicles rely on data labeling for various AI applications. - Is data labeling expensive?
Yes, depending on the volume and type of data, labeling can be resource-intensive and costly. - Can AI perform data labeling?
Yes, AI can assist in labeling; however, human verification is often required to ensure accuracy. - How is data labeling used in image recognition?
Labeling in image recognition involves marking objects within images to train models to identify similar objects. - Are there different types of data labeling?
Yes, types include image annotation, text labeling, sentiment analysis, and audio transcription. - What challenges are there in data labeling?
Challenges include maintaining accuracy, handling large volumes of data, and avoiding biases. - What tools are used for data labeling?
Common tools include Labelbox, Amazon SageMaker Ground Truth, and Dataloop.
Data Labeling Related Words
- Categories/Topics: Machine Learning, Supervised Learning, Data Annotation, Artificial Intelligence
- Word Families: Labeled, Labeler, Annotation, Tagging, Annotator
Did you know?
A pivotal moment in data labeling occurred with the release of ImageNet in 2009. This massive labeled dataset, featuring millions of images tagged for objects, revolutionized AI by providing a reliable benchmark for image recognition models.
Authors | @ArjunAndVishnu
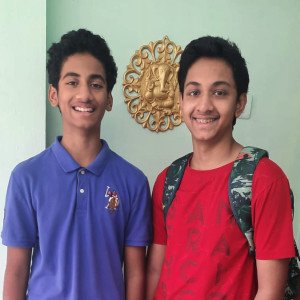
PicDictionary.com is an online dictionary in pictures. If you have questions, please reach out to us on WhatsApp or Twitter.
I am Vishnu. I like AI, Linux, Single Board Computers, and Cloud Computing. I create the web & video content, and I also write for popular websites.
My younger brother Arjun handles image & video editing. Together, we run a YouTube Channel that's focused on reviewing gadgets and explaining technology.
Comments powered by CComment