Hybrid AI
Quick Navigation:
- Hybrid AI Definition
- Hybrid AI Explained Easy
- Hybrid AI Origin
- Hybrid AI Etymology
- Hybrid AI Usage Trends
- Hybrid AI Usage
- Hybrid AI Examples in Context
- Hybrid AI FAQ
- Hybrid AI Related Words
Hybrid AI Definition
Hybrid AI combines multiple types of artificial intelligence approaches to create a more versatile, powerful, and efficient system. Rather than relying solely on a single method, such as machine learning or symbolic reasoning, Hybrid AI integrates several techniques, including rule-based systems, neural networks, and knowledge-based systems. This integration allows Hybrid AI to benefit from the strengths of each individual method while compensating for their respective weaknesses. For instance, neural networks are excellent at recognizing patterns but struggle with logical reasoning, where rule-based systems excel. Hybrid AI enables a broader range of problem-solving capabilities by merging these approaches.
Hybrid AI Explained Easy
Think of Hybrid AI like a superhero team. Each hero has unique strengths and weaknesses, and when they work together, they can solve big problems! Hybrid AI combines different types of AI “heroes,” like one that’s really good at learning patterns and another that’s good at following rules. By joining forces, Hybrid AI becomes smarter and better at solving problems than any one type of AI could on its own.
Hybrid AI Origin
The concept of Hybrid AI originated as AI researchers realized that no single approach could handle all the tasks required for complex, real-world applications. Early AI research focused on either symbolic AI, which is rule-based, or machine learning. However, the limitations of each approach led researchers to explore ways to combine them, giving rise to Hybrid AI models that integrate multiple forms of AI, particularly in fields like robotics, natural language processing, and decision-making systems.
Hybrid AI Etymology
The word "hybrid" derives from the Latin "hybrida," meaning a mix or combination, and "AI" stands for Artificial Intelligence.
Hybrid AI Usage Trends
The adoption of Hybrid AI has been steadily increasing, especially in industries that require nuanced decision-making and contextual understanding, such as healthcare, finance, and autonomous driving. Over the past decade, as more industries realize the benefits of blending different AI techniques, Hybrid AI has emerged as a practical solution for applications that demand high accuracy, adaptability, and explainability.
Hybrid AI Usage
- Formal/Technical Tagging: Integrative AI, Multi-Modal AI, Composite AI
- Typical Collocations: Hybrid AI systems, Hybrid AI models, Hybrid AI architecture, implementing Hybrid AI, leveraging Hybrid AI
Hybrid AI Examples in Context
"In healthcare, Hybrid AI systems are used to combine diagnostic image analysis with patient history data, enhancing decision-making accuracy."
"The autonomous vehicle industry leverages Hybrid AI to merge rule-based navigation with real-time data analysis, making driving safer and more efficient."
Hybrid AI FAQ
- What is Hybrid AI?
Hybrid AI is a system that combines multiple types of AI approaches to improve performance and versatility. - Why is Hybrid AI important?
It’s important because it allows AI to handle more complex tasks by combining different strengths of various AI types. - How is Hybrid AI used in healthcare?
It can analyze medical images and combine them with other patient data to improve diagnostic accuracy. - What are the main components of Hybrid AI?
Typically, it includes neural networks, rule-based systems, and sometimes, knowledge-based systems. - What industries use Hybrid AI?
Healthcare, finance, autonomous driving, and customer service often use Hybrid AI for better results. - How does Hybrid AI differ from traditional AI?
Traditional AI may use one approach, while Hybrid AI combines several, making it more adaptable. - Can Hybrid AI work with real-time data?
Yes, it’s designed to handle real-time inputs, especially in areas like autonomous vehicles. - Is Hybrid AI better than pure machine learning?
In many cases, yes, because it can solve a wider range of problems by combining different AI types. - What are the challenges of using Hybrid AI?
Integrating various AI types can be complex and resource-intensive. - Can Hybrid AI improve over time?
Yes, it can learn and adapt, especially if it includes machine learning components.
Hybrid AI Related Words
- Categories/Topics: Artificial Intelligence, Machine Learning, Robotics, Data Science
- Word Families: Hybridization, Artificial Intelligence, Integrative Learning
Did you know?
Hybrid AI has been instrumental in advancing autonomous vehicle technology. For instance, self-driving cars use a hybrid approach that combines neural networks for image recognition with rule-based systems for decision-making. This blend allows the car to "see" and interpret its environment while making real-time driving decisions. By integrating multiple AI techniques, Hybrid AI enables safer and more reliable autonomous driving, helping vehicles respond to complex road conditions and unexpected obstacles.
Authors | @ArjunAndVishnu
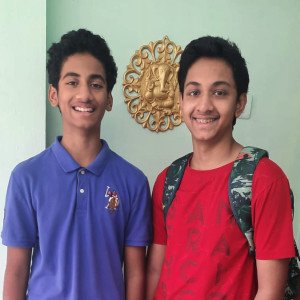
PicDictionary.com is an online dictionary in pictures. If you have questions, please reach out to us on WhatsApp or Twitter.
I am Vishnu. I like AI, Linux, Single Board Computers, and Cloud Computing. I create the web & video content, and I also write for popular websites.
My younger brother Arjun handles image & video editing. Together, we run a YouTube Channel that's focused on reviewing gadgets and explaining technology.
Comments powered by CComment