Inverse Reinforcement Learning
Quick Navigation:
- Inverse Reinforcement Learning Definition
- Inverse Reinforcement Learning Explained Easy
- Inverse Reinforcement Learning Origin
- Inverse Reinforcement Learning Etymology
- Inverse Reinforcement Learning Usage Trends
- Inverse Reinforcement Learning Usage
- Inverse Reinforcement Learning Examples in Context
- Inverse Reinforcement Learning FAQ
- Inverse Reinforcement Learning Related Words
Inverse Reinforcement Learning Definition
Inverse Reinforcement Learning (IRL) is a subset of machine learning focused on discovering a reward function based on observing behavior from an expert or agent. Instead of directly optimizing a given reward function (as in traditional reinforcement learning), IRL deduces the reward structure that would likely lead to observed actions. This method is invaluable in settings where the optimal behavior is known, but the exact reward function driving it is not, making it suitable for complex tasks such as human behavior modeling, autonomous driving, and robotics.
Inverse Reinforcement Learning Explained Easy
Imagine you’re watching a skilled chess player and trying to figure out their strategy. You don’t know their exact goals, but by observing their moves, you can guess what they’re trying to achieve. Inverse Reinforcement Learning works similarly: it looks at someone’s actions to understand the reward or goal they’re aiming for.
Inverse Reinforcement Learning Origin
The origins of IRL lie in the advancements of reinforcement learning and behavioral psychology. As researchers sought methods to train AI without explicitly defining all rewards, IRL emerged as a natural approach to learning by example, becoming prominent in robotics and AI applications.
Inverse Reinforcement Learning Etymology
The term "inverse" highlights that IRL reverses the typical reinforcement learning process: instead of starting with a reward to guide actions, it starts with actions to infer rewards.
Inverse Reinforcement Learning Usage Trends
Interest in IRL has surged alongside the development of AI and robotics. Its application in autonomous vehicles, healthcare robotics, and behavior prediction models continues to grow, fueled by the need for AI systems that can learn goals from expert demonstrations rather than predefined rewards.
Inverse Reinforcement Learning Usage
- Formal/Technical Tagging:
- Machine Learning
- Artificial Intelligence
- Behavioral Modeling - Typical Collocations:
- "inverse reinforcement learning algorithm"
- "reward function inference"
- "learning from expert behavior"
- "IRL applications in robotics"
Inverse Reinforcement Learning Examples in Context
- Inverse Reinforcement Learning can help autonomous vehicles learn driving strategies by observing experienced drivers.
- Healthcare robotics use IRL to learn ideal patient interactions by studying expert healthcare providers.
- IRL models in AI can predict customer behavior by analyzing past actions, allowing personalized recommendations.
Inverse Reinforcement Learning FAQ
- What is Inverse Reinforcement Learning?
Inverse Reinforcement Learning (IRL) is a learning approach where a model deduces a reward function based on observed actions of an expert or agent. - How does IRL differ from traditional reinforcement learning?
IRL infers the reward structure based on observed actions, while reinforcement learning typically optimizes a known reward function. - What are common applications of IRL?
IRL is used in autonomous driving, robotics, behavior prediction, and situations where reward structures aren’t directly defined. - Why is IRL useful in AI?
It allows AI to learn from demonstrations without explicit reward definitions, ideal for complex environments. - Can IRL be applied to human behavior modeling?
Yes, IRL is widely used to model human actions and predict decisions based on past behaviors. - What challenges does IRL face?
Challenges include computational complexity and accurately capturing nuanced behavior from demonstrations. - Is IRL commonly used in robotics?
Yes, especially for tasks that involve human-robot interaction or autonomous navigation. - How does IRL benefit autonomous vehicles?
IRL helps vehicles learn safe driving techniques by observing human drivers. - Can IRL improve healthcare robotics?
Yes, IRL enables healthcare robots to learn best practices by observing expert healthcare workers. - What fields are currently advancing IRL research?
Fields like autonomous systems, human-AI interaction, and behavior prediction are actively advancing IRL.
Inverse Reinforcement Learning Related Words
- Categories/Topics:
- Machine Learning
- Robotics
- Human Behavior Modeling
Did you know?
Inverse Reinforcement Learning has become integral to creating more adaptive and intuitive AI systems. Notably, it’s used in robotics to teach robots complex tasks like assisting the elderly by observing human caregivers, allowing robots to act with empathy and sensitivity in real-world environments.
Authors | @ArjunAndVishnu
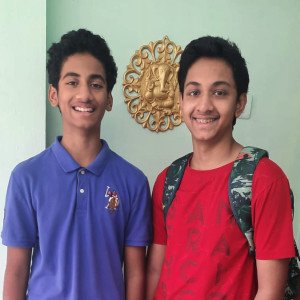
PicDictionary.com is an online dictionary in pictures. If you have questions, please reach out to us on WhatsApp or Twitter.
I am Vishnu. I like AI, Linux, Single Board Computers, and Cloud Computing. I create the web & video content, and I also write for popular websites.
My younger brother Arjun handles image & video editing. Together, we run a YouTube Channel that's focused on reviewing gadgets and explaining technology.
Comments powered by CComment