Question Answering (QA)
Quick Navigation:
- Question Answering Definition
- Question Answering Explained Easy
- Question Answering Origin
- Question Answering Etymology
- Question Answering Usage Trends
- Question Answering Usage
- Question Answering Examples in Context
- Question Answering FAQ
- Question Answering Related Words
Question Answering Definition
Question Answering in AI involves using models and algorithms to respond accurately to user queries. It requires a combination of natural language understanding, retrieval of relevant information, and response generation. These systems range from simple FAQ-style answers to sophisticated dialogue models that provide insightful responses. In AI-driven applications, Question Answering plays a vital role in areas like search engines, chatbots, and customer support, using techniques such as retrieval-based methods, transformer models, and deep learning to enhance response accuracy.
Question Answering Explained Easy
Imagine you ask a librarian any question, and she quickly finds you the right book with the answer. AI Question Answering systems are like that librarian, but faster. When you ask a question, they look through tons of information and give you the best answer.
Question Answering Origin
The development of Question Answering systems traces back to the early days of artificial intelligence and information retrieval. Early research in the 1960s focused on database-style querying systems. With advancements in natural language processing and machine learning, Question Answering evolved into a complex field capable of handling open-ended questions across diverse domains.
Question Answering Etymology
The term “Question Answering” is straightforward, reflecting the function of the system: to answer questions posed by users accurately and efficiently.
Question Answering Usage Trends
Recently, Question Answering systems have surged in popularity, largely due to advancements in NLP and machine learning. As businesses recognize the value in automating responses, industries like e-commerce, healthcare, and finance are increasingly adopting these systems for chatbots, virtual assistants, and knowledge bases. The rise of conversational AI has further driven interest in QA systems, making them essential in user engagement and support.
Question Answering Usage
- Formal/Technical Tagging:
- Natural Language Processing (NLP)
- Machine Learning
- Conversational AI - Typical Collocations:
- "Question Answering model"
- "Question Answering system"
- "automated Question Answering"
- "knowledge retrieval in Question Answering"
Question Answering Examples in Context
- An AI-powered Question Answering system can instantly answer customer inquiries on an e-commerce platform.
- Virtual assistants like Siri and Alexa rely on Question Answering models to respond to user questions accurately.
- In educational platforms, Question Answering systems assist students by providing precise answers to learning queries.
Question Answering FAQ
- What is Question Answering in AI?
Question Answering is a technology in AI that generates responses to questions by retrieving relevant information and creating accurate answers. - How does Question Answering work?
It combines NLP techniques to understand questions, retrieves information, and formulates answers. - Is Question Answering different from search engines?
Yes, while search engines return links, Question Answering provides direct answers. - What are the main types of Question Answering systems?
There are retrieval-based and generative Question Answering systems. - How is Question Answering used in virtual assistants?
It powers voice assistants by interpreting questions and providing relevant responses. - Can Question Answering be used in healthcare?
Yes, it assists doctors and patients by providing medical information and answering common health questions. - What models are used for Question Answering?
Models like BERT, GPT, and T5 are popular for developing QA systems. - What is the challenge in building Question Answering systems?
Challenges include understanding complex questions, context handling, and providing accurate responses. - Why is NLP important for Question Answering?
NLP enables the understanding and processing of human language, crucial for generating accurate answers. - How does Question Answering benefit businesses?
It enhances customer engagement, reduces support costs, and improves accessibility to information.
Question Answering Related Words
- Categories/Topics:
- Natural Language Processing
- Artificial Intelligence
- Knowledge Retrieval
Did you know?
Question Answering has been instrumental in the development of open-domain question answering (ODQA), a challenging field where AI systems answer questions without relying on a specific dataset. By training on vast text data, these systems can answer a wide variety of questions, significantly improving user interaction in open-ended domains.
Authors | @ArjunAndVishnu
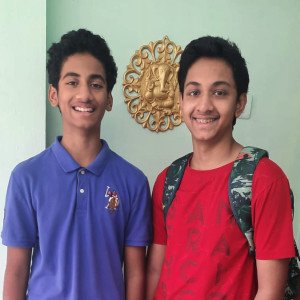
PicDictionary.com is an online dictionary in pictures. If you have questions, please reach out to us on WhatsApp or Twitter.
I am Vishnu. I like AI, Linux, Single Board Computers, and Cloud Computing. I create the web & video content, and I also write for popular websites.
My younger brother Arjun handles image & video editing. Together, we run a YouTube Channel that's focused on reviewing gadgets and explaining technology.
Comments powered by CComment