Visual Anomaly Detection
Quick Navigation:
- Visual Anomaly Detection Definition
- Visual Anomaly Detection Explained Easy
- Visual Anomaly Detection Origin
- Visual Anomaly Detection Etymology
- Visual Anomaly Detection Usage Trends
- Visual Anomaly Detection Usage
- Visual Anomaly Detection Examples in Context
- Visual Anomaly Detection FAQ
- Visual Anomaly Detection Related Words
Visual Anomaly Detection Definition
Visual Anomaly Detection is an AI-based technique used to identify patterns, objects, or events that deviate from expected visual norms. This technique leverages machine learning algorithms and computer vision to analyze images or videos for anomalies, often used in quality control, security, and healthcare. In manufacturing, it spots defective products by comparing them to known standards, and in healthcare, it aids in diagnosing conditions by detecting irregularities in medical images. By training models on a wide array of visual data, Visual Anomaly Detection improves accuracy and efficiency in automated inspection tasks.
Visual Anomaly Detection Explained Easy
Think of Visual Anomaly Detection like a tool that checks if everything looks the way it should. Imagine you’re sorting apples, and you know what a good apple should look like. This tool is like a super-powered machine that checks each apple, finding the ones that look strange or have spots. It’s smart enough to tell a good apple from a bad one by remembering what it’s learned before.
Visual Anomaly Detection Origin
The concept of Visual Anomaly Detection evolved as a subset of anomaly detection in AI, focusing on visual data analysis. This specialization developed alongside advances in computer vision and neural networks, becoming especially prominent in the early 21st century as image-based AI applications grew in precision and reliability.
Visual Anomaly Detection Etymology
The term "visual anomaly detection" combines "visual," relating to images and vision, with "anomaly detection," a phrase indicating the identification of unexpected or rare items or patterns.
Visual Anomaly Detection Usage Trends
Visual Anomaly Detection is rapidly expanding across sectors like manufacturing, healthcare, and security. Its ability to automate visual inspections and improve quality control has led to high adoption in production lines and medical diagnostics, especially with increased reliance on digital and remote inspections. As the demand for efficient, automated solutions grows, Visual Anomaly Detection remains a key technology in AI-driven operational processes.
Visual Anomaly Detection Usage
- Formal/Technical Tagging:
- Machine Learning
- Computer Vision
- Quality Control
- Predictive Maintenance - Typical Collocations:
- "visual anomaly detection system"
- "automated quality inspection"
- "AI-based defect detection"
- "real-time visual anomaly detection"
Visual Anomaly Detection Examples in Context
- A visual anomaly detection system on an automotive production line identifies paint defects on car parts.
- In healthcare, visual anomaly detection helps identify potential tumors in medical scans.
- Security systems employ visual anomaly detection to flag suspicious activities in surveillance footage.
Visual Anomaly Detection FAQ
- What is Visual Anomaly Detection?
Visual Anomaly Detection is an AI method that identifies unusual patterns or items in visual data, such as images or video. - How is Visual Anomaly Detection used in manufacturing?
It automates the process of spotting defects in products, ensuring higher quality control standards. - Can Visual Anomaly Detection work in real time?
Yes, modern systems can analyze visual data in real time, often used in surveillance and safety applications. - What role does Visual Anomaly Detection play in healthcare?
It assists in diagnosing conditions by spotting anomalies in medical images, enhancing diagnostic accuracy. - Is Visual Anomaly Detection only applicable to images?
Primarily, yes, but it can also be used in video streams, especially for live surveillance. - How accurate is Visual Anomaly Detection?
Accuracy depends on the quality of the training data and model used but can reach high levels with refined models. - What are common algorithms in Visual Anomaly Detection?
Algorithms include convolutional neural networks (CNNs) and generative adversarial networks (GANs). - How does it differ from traditional anomaly detection?
Visual Anomaly Detection specifically targets visual data, while traditional methods may focus on numerical or categorical data. - Why is it useful in predictive maintenance?
By spotting early signs of wear or defects, it helps schedule maintenance before failures occur. - How does Visual Anomaly Detection help in quality control?
It automates defect detection, reducing manual inspection needs and increasing consistency.
Visual Anomaly Detection Related Words
- Categories/Topics:
- Machine Learning
- Anomaly Detection
- Computer Vision
- Healthcare AI
Did you know?
Visual Anomaly Detection is revolutionizing satellite imaging analysis by helping detect environmental changes, such as deforestation or pollution levels, with remarkable accuracy. This technology allows scientists to monitor vast areas with minimal human intervention, enabling quicker responses to ecological threats.
Authors | @ArjunAndVishnu
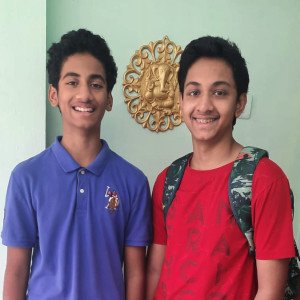
PicDictionary.com is an online dictionary in pictures. If you have questions, please reach out to us on WhatsApp or Twitter.
I am Vishnu. I like AI, Linux, Single Board Computers, and Cloud Computing. I create the web & video content, and I also write for popular websites.
My younger brother Arjun handles image & video editing. Together, we run a YouTube Channel that's focused on reviewing gadgets and explaining technology.
Comments powered by CComment