Capsule Networks
Quick Navigation:
- Capsule Networks Definition
- Capsule Networks Explained Easy
- Capsule Networks Origin
- Capsule Networks Etymology
- Capsule Networks Usage Trends
- Capsule Networks Usage
- Capsule Networks Examples in Context
- Capsule Networks FAQ
- Capsule Networks Related Words
Capsule Networks Definition
Capsule Networks are an advanced type of artificial neural network introduced to address limitations in traditional convolutional neural networks (CNNs). Unlike CNNs, which use pooling layers that often lose valuable spatial information, Capsule Networks maintain spatial hierarchies, allowing the network to better understand the relationships between objects in an image. Capsules are specialized groups of neurons that preserve detailed data on an object’s position, rotation, and other features, enhancing model accuracy on tasks requiring an understanding of 3D structures.
Capsule Networks Explained Easy
Imagine a stack of building blocks that need to be arranged in a specific shape. If one block is slightly off, the structure doesn’t look right. Capsule Networks work like a careful builder who can check each block's position and rotation to create the perfect shape. They help computers understand where things are and how they’re shaped, making sure the “image” they see looks as close to real life as possible.
Capsule Networks Origin
Capsule Networks were introduced by Geoffrey Hinton and his team in 2017 as a solution to some of the limitations of convolutional neural networks (CNNs). Hinton, often referred to as the "Godfather of Deep Learning," identified that CNNs lose spatial information through pooling, prompting the development of Capsule Networks for better handling of spatial relationships in images.
Capsule Networks Etymology
The term "capsule" in Capsule Networks refers to a group of neurons working together to capture and preserve detailed spatial and pose information about objects in an image.
Capsule Networks Usage Trends
Since their introduction, Capsule Networks have gained attention in fields requiring precise image recognition, such as medical imaging and autonomous driving. Although CNNs remain widely used, the focus on spatial relationships in Capsule Networks has sparked interest in industries where identifying complex shapes and objects is essential. The adoption rate, however, is still growing as more computational power and optimization techniques are needed.
Capsule Networks Usage
- Formal/Technical Tagging:
- Neural Networks
- Deep Learning
- Image Recognition - Typical Collocations:
- "Capsule Network model"
- "routing between capsules"
- "Capsule Networks in image processing"
- "multi-layer capsules"
Capsule Networks Examples in Context
- Capsule Networks have shown promising results in diagnosing diseases from medical scans, where understanding fine details is crucial.
- Autonomous vehicle systems use Capsule Networks to detect obstacles with better precision by understanding the 3D structure of objects.
- Capsule Networks enhance facial recognition technologies by recognizing subtle facial features, improving accuracy over traditional CNNs.
Capsule Networks FAQ
- What is a Capsule Network?
A Capsule Network is a neural network architecture that preserves spatial hierarchies, making it better at understanding 3D structures than traditional CNNs. - How do Capsule Networks differ from CNNs?
Capsule Networks maintain spatial information through “capsules” of neurons, avoiding the pooling layers that often lose details in CNNs. - What are some applications of Capsule Networks?
Capsule Networks are used in fields like medical imaging, autonomous driving, and facial recognition, where spatial accuracy is essential. - Who developed Capsule Networks?
Geoffrey Hinton and his team introduced Capsule Networks in 2017. - What does “routing between capsules” mean?
It refers to the mechanism Capsule Networks use to determine how data flows between capsules, allowing the network to learn spatial relationships more accurately. - Are Capsule Networks better than CNNs?
Capsule Networks excel in tasks needing spatial awareness, though they require more computational resources than CNNs. - Why aren’t Capsule Networks widely used yet?
They require significant computational power and are less optimized than CNNs, limiting their broad adoption. - Can Capsule Networks improve medical diagnostics?
Yes, Capsule Networks are effective in medical imaging for detecting intricate patterns in scans. - What fields are adopting Capsule Networks?
Capsule Networks are finding applications in healthcare, autonomous vehicles, and high-precision image recognition. - Are Capsule Networks suitable for real-time applications?
Due to their high computational demands, Capsule Networks are challenging to use in real-time settings but hold potential as technology advances.
Capsule Networks Related Words
- Categories/Topics:
- Machine Learning
- Neural Networks
- Image Processing
Did you know?
Capsule Networks can recognize objects regardless of their orientation. This capability is valuable in robotic vision, as robots need to understand objects from various angles, making Capsule Networks an exciting development in AI-driven robotics.
Authors | Arjun Vishnu | @ArjunAndVishnu
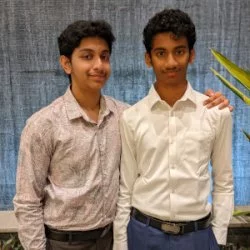
PicDictionary.com is an online dictionary in pictures. If you have questions or suggestions, please reach out to us on WhatsApp or Twitter.
I am Vishnu. I like AI, Linux, Single Board Computers, and Cloud Computing. I create the web & video content, and I also write for popular websites.
My younger brother, Arjun handles image & video editing. Together, we run a YouTube Channel that's focused on reviewing gadgets and explaining technology.
Comments (0)
Comments powered by CComment