Bias Mitigation
Quick Navigation:
- Bias Mitigation Definition
- Bias Mitigation Explained Easy
- Bias Mitigation Origin
- Bias Mitigation Etymology
- Bias Mitigation Usage Trends
- Bias Mitigation Usage
- Bias Mitigation Examples in Context
- Bias Mitigation FAQ
- Bias Mitigation Related Words
Bias Mitigation Definition
Bias mitigation in AI refers to the strategies and techniques employed to reduce or eliminate biased outcomes in machine learning models and algorithms. This involves adjusting data, refining model training processes, and implementing safeguards to ensure fair, accurate, and ethical AI systems. Effective bias mitigation considers the diverse impacts of AI on different communities and aims to reduce systemic unfairness and prejudice in automated decisions.
Bias Mitigation Explained Easy
Think of it like this: imagine you’re making a list of your favorite foods, but you only ask your friends who love pizza. Your list will be biased towards pizza lovers! Bias mitigation is like asking lots of different people about their favorite foods to make sure everyone’s tastes are considered.
Bias Mitigation Origin
The concept of bias mitigation emerged from concerns about fairness in decision-making systems, especially as AI started being used in critical fields like hiring, healthcare, and law. Researchers began studying and addressing bias in algorithms to prevent discrimination and ensure equitable outcomes.
Bias Mitigation Etymology
The term "bias mitigation" stems from "bias," meaning prejudice or inclination, and "mitigation," which means reducing the severity or seriousness of something.
Bias Mitigation Usage Trends
Bias mitigation has grown in importance as AI becomes more prevalent. With increased awareness of algorithmic bias, especially in fields affecting human welfare and rights, tech companies, policymakers, and researchers are investing heavily in methods to mitigate bias, pushing for AI ethics and fairness regulations worldwide.
Bias Mitigation Usage
- Formal/Technical Tagging:
- Fairness
- Algorithmic Ethics
- Responsible AI - Typical Collocations:
- "bias mitigation techniques"
- "ethical AI practices"
- "algorithm bias"
- "bias reduction methods"
Bias Mitigation Examples in Context
- Bias mitigation techniques are applied to hiring algorithms to prevent gender or racial discrimination.
- In healthcare, bias mitigation ensures that medical AI tools do not favor certain demographics over others.
- Social media companies use bias mitigation in content algorithms to promote diverse viewpoints.
Bias Mitigation FAQ
- What is bias mitigation?
Bias mitigation is the process of reducing or eliminating biases in AI systems to promote fairness and accuracy. - Why is bias mitigation important?
It prevents AI systems from unfairly favoring or discriminating against individuals or groups. - How is bias mitigation achieved in AI?
Through data balancing, fairness-aware algorithms, and ethical oversight. - Can bias be completely eliminated?
It is challenging, but effective bias mitigation can greatly reduce bias and its impact. - What role does data play in bias mitigation?
Diverse, representative data is crucial for reducing bias in AI models. - Are there regulations on AI bias?
Many regions are introducing or exploring regulations for fair and ethical AI practices. - What industries prioritize bias mitigation?
Healthcare, finance, education, and hiring are heavily focused on bias mitigation. - How do tech companies address AI bias?
By implementing fairness-focused research, guidelines, and tools. - Does bias mitigation affect AI accuracy?
It can, but proper techniques aim to improve both fairness and accuracy. - What challenges exist in bias mitigation?
Challenges include identifying biases, balancing diverse needs, and evolving regulations.
Bias Mitigation Related Words
- Categories/Topics:
- AI Ethics
- Algorithmic Fairness
- Social Impact
Did you know?
In 2020, a major tech company launched a fairness toolkit to detect and mitigate bias across its AI systems. This toolkit has since become a benchmark for AI bias detection and is widely used in academia and industry to promote equitable machine learning practices.
Authors | @ArjunAndVishnu
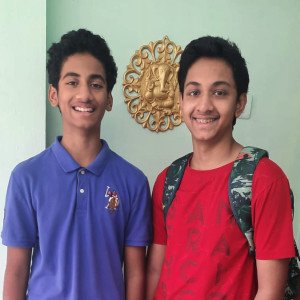
PicDictionary.com is an online dictionary in pictures. If you have questions, please reach out to us on WhatsApp or Twitter.
I am Vishnu. I like AI, Linux, Single Board Computers, and Cloud Computing. I create the web & video content, and I also write for popular websites.
My younger brother Arjun handles image & video editing. Together, we run a YouTube Channel that's focused on reviewing gadgets and explaining technology.
Comments powered by CComment