Neuromorphic Computing
Quick Navigation:
- Neuromorphic Computing Definition
- Neuromorphic Computing Explained Easy
- Neuromorphic Computing Origin
- Neuromorphic Computing Etymology
- Neuromorphic Computing Usage Trends
- Neuromorphic Computing Usage
- Neuromorphic Computing Examples in Context
- Neuromorphic Computing FAQ
- Neuromorphic Computing Related Words
Neuromorphic Computing Definition
Neuromorphic computing is an innovative approach in artificial intelligence, where computational hardware mimics the brain's neural structures. Utilizing specialized circuits and systems, this technology enables complex processing with lower power consumption, ideal for AI tasks that require real-time adaptability, such as sensory processing and pattern recognition. Neuromorphic chips incorporate artificial neurons and synapses that communicate via spiking signals, resembling brain activity, which allows more efficient parallel processing and learning over traditional computing methods.
Neuromorphic Computing Explained Easy
Think of a computer that tries to work like your brain. Instead of processing one thing at a time, like normal computers, it can do many things at once, using parts that act like brain cells. This way, it’s faster and uses less energy, just like our brain does when we recognize sounds or images.
Neuromorphic Computing Origin
The field of neuromorphic computing emerged in the 1980s, with a growing interest in creating machines that mimic the brain's efficiency and flexibility. Research in neuroscience and semiconductor technology converged, leading to hardware designed to emulate brain-like functions. It has since become a core area in advanced AI research.
Neuromorphic Computing Etymology
The term “neuromorphic” derives from Greek roots "neuro," meaning “nerve,” and "morph," meaning “form” or “shape.” It refers to creating systems that resemble the brain's structure.
Neuromorphic Computing Usage Trends
Neuromorphic computing has gained momentum due to the rise in demand for AI applications that need low-latency and energy-efficient processing, such as autonomous vehicles, robotics, and real-time data analysis in edge devices. Its ability to support adaptive learning without needing cloud-based resources makes it particularly valuable in decentralized applications.
Neuromorphic Computing Usage
- Formal/Technical Tagging: Machine Learning, Brain-inspired Computing, Edge Computing
- Typical Collocations: "neuromorphic chip," "spiking neural network," "brain-inspired computing hardware," "low-power neuromorphic processing"
Neuromorphic Computing Examples in Context
- Neuromorphic computing enables robots to process sensory data in real-time, mimicking human reflexes.
- Smartphones equipped with neuromorphic processors can recognize gestures or voices with minimal battery usage.
- Medical devices use neuromorphic computing to analyze neural signals for monitoring health conditions.
Neuromorphic Computing FAQ
- What is neuromorphic computing?
Neuromorphic computing involves creating computer systems that work like the human brain, making them more efficient for certain AI tasks. - How does neuromorphic computing differ from traditional computing?
Neuromorphic computing uses brain-inspired architectures, allowing parallel processing and reduced energy use, unlike traditional sequential computing. - What is a spiking neural network?
It’s a type of neural network that sends signals between artificial neurons, similar to how neurons communicate in the brain. - What are some applications of neuromorphic computing?
Applications include robotics, autonomous vehicles, medical devices, and low-power edge computing. - Why is neuromorphic computing important in AI?
It supports AI with high efficiency and low power needs, critical for real-time and decentralized applications. - How do neuromorphic chips work?
Neuromorphic chips use artificial neurons and synapses to process data through "spikes" or electrical pulses, much like the brain. - Can neuromorphic computing be used in smart devices?
Yes, it is ideal for smart devices, enabling low-energy processing for tasks like voice or gesture recognition. - Is neuromorphic computing scalable?
Neuromorphic designs are scalable and can be integrated into various devices, from small sensors to large-scale systems. - How does neuromorphic computing benefit edge computing?
It allows data processing at the source (edge), reducing latency and energy consumption compared to cloud-based processing. - Are there commercial products using neuromorphic computing?
Yes, companies have developed neuromorphic processors for AI-driven applications in robotics, healthcare, and smart devices.
Neuromorphic Computing Related Words
- Categories/Topics: Machine Learning, Brain-inspired Computing, Artificial Intelligence, Edge Computing
Did you know? In 2020, IBM developed a neuromorphic processor capable of running complex neural network models while consuming 100 times less power than conventional chips. This breakthrough has paved the way for energy-efficient AI in many industries, from healthcare to autonomous robotics.
Authors | Arjun Vishnu | @ArjunAndVishnu
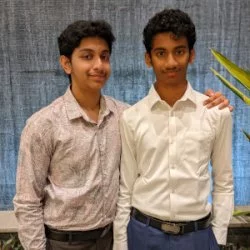
PicDictionary.com is an online dictionary in pictures. If you have questions or suggestions, please reach out to us on WhatsApp or Twitter.
I am Vishnu. I like AI, Linux, Single Board Computers, and Cloud Computing. I create the web & video content, and I also write for popular websites.
My younger brother, Arjun handles image & video editing. Together, we run a YouTube Channel that's focused on reviewing gadgets and explaining technology.
Comments (0)
Comments powered by CComment