ETL (Extract, Transform, Load)
(Representational Image | Source: Dall-E)
Quick Navigation:
- ETL Definition
- ETL Explained Easy
- ETL Origin
- ETL Etymology
- ETL Usage Trends
- ETL Usage
- ETL Examples in Context
- ETL FAQ
- ETL Related Words
ETL Definition
ETL (Extract, Transform, Load) is a data integration process that collects data from various sources, modifies it into a usable format, and then loads it into a target system such as a database or data warehouse. The Extract phase gathers raw data from multiple structured and unstructured sources. The Transform phase cleanses, standardizes, and processes the data according to business rules. The Load phase ensures the transformed data is stored in a structured format for analytics, reporting, and decision-making. ETL is widely used in business intelligence, data warehousing, and big data analytics.
ETL Explained Easy
Imagine you have a messy pile of Lego bricks from different sets. Extracting is like gathering all the Lego bricks from around the room. Transforming is when you sort them by size and color, remove broken pieces, and assemble them into a new structure. Loading is like placing your finished Lego creation onto a display shelf. ETL does something similar with data—it collects, cleans, and organizes information so businesses can use it effectively.
ETL Origin
ETL emerged in the 1970s as organizations sought ways to integrate data from multiple systems into centralized databases. With the rise of relational databases in the 1980s, ETL processes became essential for managing enterprise data. The demand for ETL grew further with the rise of data warehouses in the 1990s and big data platforms in the 2000s.
ETL Etymology
The term ETL originates from the three distinct steps involved in the process: Extract from Latin extrahere (to draw out), Transform from Latin transformare (to change in shape or nature), and Load from Old English hladan (to put or place into).
ETL Usage Trends
ETL has evolved significantly with cloud computing, real-time processing, and AI-driven automation. Traditional batch-processing ETL is now complemented by ELT (Extract, Load, Transform), where transformation occurs in the target database. Modern ETL tools also integrate with data lakes, making them more scalable for big data applications.
ETL Usage
- Formal/Technical Tagging:
- Data Integration
- Data Warehousing
- Business Intelligence - Typical Collocations:
- "ETL pipeline"
- "ETL data processing"
- "automated ETL workflows"
- "ETL tools for data integration"
ETL Examples in Context
- A financial institution extracts transaction records, cleans them by removing duplicates, and loads them into a data warehouse for fraud detection.
- An e-commerce company uses ETL to gather sales data, normalize product categories, and store it in a structured format for reporting.
- A healthcare provider extracts patient records from multiple systems, transforms them into a standardized format, and loads them into an analytics platform for medical research.
ETL FAQ
- What is ETL used for?
ETL is used for data integration, enabling businesses to consolidate, clean, and analyze data from different sources. - How does ETL differ from ELT?
In ETL, data transformation occurs before loading, whereas in ELT, data is first loaded into a storage system and then transformed. - What are the key components of an ETL pipeline?
The key components include extraction from data sources, transformation using business rules, and loading into a target system. - Which industries rely on ETL?
ETL is crucial in finance, healthcare, retail, telecommunications, and many other industries requiring data management. - What tools are commonly used for ETL?
Popular ETL tools include Apache Nifi, Talend, Informatica, Microsoft SSIS, and AWS Glue. - Can ETL be used for real-time data processing?
Traditional ETL is batch-oriented, but modern ETL tools support real-time streaming data processing. - What are the challenges of ETL?
ETL faces challenges like handling large datasets, ensuring data quality, and optimizing performance for speed and efficiency. - Is ETL necessary for machine learning?
Yes, ETL is critical for preparing structured and clean data required for machine learning models. - How does ETL benefit business intelligence?
ETL enables data-driven decision-making by organizing and structuring raw data for analytics. - Are there cloud-based ETL solutions?
Yes, cloud-based ETL solutions like Google Cloud Dataflow, AWS Glue, and Azure Data Factory offer scalable and flexible data integration.
ETL Related Words
- Categories/Topics:
- Data Engineering
- Business Intelligence
- Big Data Analytics
Did you know?
One of the earliest large-scale ETL implementations was in the airline industry, where airlines consolidated ticket sales, flight schedules, and customer data from various booking systems into centralized databases, laying the foundation for data-driven decision-making.
Authors | Arjun Vishnu | @ArjunAndVishnu
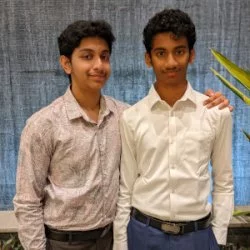
PicDictionary.com is an online dictionary in pictures. If you have questions or suggestions, please reach out to us on WhatsApp or Twitter.
I am Vishnu. I like AI, Linux, Single Board Computers, and Cloud Computing. I create the web & video content, and I also write for popular websites.
My younger brother, Arjun handles image & video editing. Together, we run a YouTube Channel that's focused on reviewing gadgets and explaining technology.
Comments (0)
Comments powered by CComment