Double Descent
Quick Navigation:
- Double Descent Definition
- Double Descent Explained Easy
- Double Descent Origin
- Double Descent Etymology
- Double Descent Usage Trends
- Double Descent Usage
- Double Descent Examples in Context
- Double Descent FAQ
- Double Descent Related Words
Double Descent Definition
Double Descent is a phenomenon in machine learning where, as model complexity increases, prediction error initially decreases, then unexpectedly increases, only to decrease again beyond a certain threshold. This effect challenges the conventional bias-variance tradeoff in statistical learning, as it suggests that higher model complexity can lead to improved accuracy when enough data is available. Double Descent typically occurs in deep neural networks and other high-capacity models, revealing unique behavior in how models generalize from training to unseen data.
Double Descent Explained Easy
Imagine building a tower with blocks. At first, adding blocks makes the tower strong. But if you keep stacking too many, the tower wobbles and falls. If you keep going, however, you may reach a point where it becomes stable again. Double Descent is similar in machine learning: as we add more complexity, performance improves, worsens, and then surprisingly improves again.
Double Descent Origin
The study of Double Descent began in the early 2000s, but it gained traction with the rise of deep learning. Researchers noticed models performing better at higher complexities, sparking new investigations into training dynamics and model capacity.
Double Descent Etymology
The term "Double Descent" refers to the pattern of error rates "descending" twice, once with initial complexity and again as complexity surpasses a critical point.
Double Descent Usage Trends
Double Descent has seen increasing attention in AI research, especially as models grow in size and capability. With deep learning architectures, this phenomenon has become relevant for performance optimization and understanding model generalization, making it an area of active study in academia and industry.
Double Descent Usage
- Formal/Technical Tagging:
Machine Learning, AI, Generalization, Model Complexity - Typical Collocations:
"Double Descent curve," "neural network Double Descent," "training complexity Double Descent," "Double Descent generalization"
Double Descent Examples in Context
- In image recognition, Double Descent can lead to improved accuracy with larger, complex models.
- Some language models benefit from Double Descent, improving performance as more layers are added.
- Double Descent explains why some high-capacity models perform better with over-parameterization.
Double Descent FAQ
- What is Double Descent in machine learning?
Double Descent is a phenomenon where model error decreases, then increases, and decreases again as model complexity grows. - How does Double Descent differ from bias-variance tradeoff?
Unlike bias-variance, which suggests error increases with over-complexity, Double Descent shows error can reduce with further complexity. - Where is Double Descent commonly observed?
In deep neural networks and high-capacity models that handle complex data. - Does Double Descent apply to all machine learning models?
No, it mainly appears in models with sufficient capacity and data. - Why does Double Descent happen?
It’s thought to result from interactions between model capacity, data quantity, and over-parameterization. - Is Double Descent beneficial?
Yes, it can improve model performance if complexity is carefully managed. - How is Double Descent useful in AI?
It helps refine training techniques and understand model behavior with complex architectures. - Is Double Descent predictable?
It’s challenging to predict precisely but is observed in high-complexity model settings. - Does Double Descent affect interpretability?
Higher complexity can reduce interpretability, making Double Descent models harder to analyze. - Can Double Descent be mitigated?
Techniques like regularization and balanced complexity can influence the effect.
Double Descent Related Words
- Categories/Topics:
Machine Learning, Deep Learning, Model Optimization, Overfitting, Generalization
Did you know?
Double Descent was first observed with simple polynomial models but gained widespread attention through deep learning, where models with extreme parameters unexpectedly yielded better results. This reshaped how researchers think about training and model capacity in AI.
Authors | Arjun Vishnu | @ArjunAndVishnu
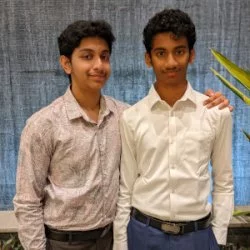
PicDictionary.com is an online dictionary in pictures. If you have questions or suggestions, please reach out to us on WhatsApp or Twitter.
I am Vishnu. I like AI, Linux, Single Board Computers, and Cloud Computing. I create the web & video content, and I also write for popular websites.
My younger brother, Arjun handles image & video editing. Together, we run a YouTube Channel that's focused on reviewing gadgets and explaining technology.
Comments (0)
Comments powered by CComment