Deep Convolutional Generative Adversarial Network (DCGAN)
Quick Navigation:
- Deep Convolutional Generative Adversarial Network Definition
- Deep Convolutional Generative Adversarial Network Explained Easy
- Deep Convolutional Generative Adversarial Network Origin
- Deep Convolutional Generative Adversarial Network Etymology
- Deep Convolutional Generative Adversarial Network Usage Trends
- Deep Convolutional Generative Adversarial Network Usage
- Deep Convolutional Generative Adversarial Network Examples in Context
- Deep Convolutional Generative Adversarial Network FAQ
- Deep Convolutional Generative Adversarial Network Related Words
Deep Convolutional Generative Adversarial Network Definition
A Deep Convolutional Generative Adversarial Network (DCGAN) is an architecture that combines deep convolutional layers with adversarial training, creating highly realistic image generation models. DCGANs involve two neural networks: a generator, which creates images from random noise, and a discriminator, which evaluates image authenticity. Through iterative training, both networks refine their functions, achieving realistic outputs. DCGANs are instrumental in applications such as art generation, video game assets, and medical imaging.
Deep Convolutional Generative Adversarial Network Explained Easy
Imagine you’re trying to make a perfect drawing while competing with a friend who points out flaws. Over time, you get better as your friend keeps challenging you. DCGANs work similarly: one network creates images, while another decides if they look real. They compete and improve, leading to lifelike creations.
Deep Convolutional Generative Adversarial Network Origin
DCGANs emerged as an extension of GANs (Generative Adversarial Networks), pioneered by Ian Goodfellow in 2014. The introduction of convolutional layers into GANs provided structure, leading to the creation of DCGANs, which excel in image-related tasks.
Deep Convolutional Generative Adversarial Network Etymology
The term “DCGAN” integrates "deep" for deep learning and "convolutional" from convolutional neural networks, denoting the specific layers that enhance image processing within the GAN structure.
Deep Convolutional Generative Adversarial Network Usage Trends
DCGANs have gained immense popularity as tools for content creation, particularly in industries like gaming, media, and virtual reality. With enhanced computational resources, DCGANs have become central to AI research, empowering both research projects and commercial applications.
Deep Convolutional Generative Adversarial Network Usage
- Formal/Technical Tagging:
- Machine Learning
- Deep Learning
- Generative Modeling - Typical Collocations:
- "DCGAN architecture"
- "training DCGAN models"
- "image generation with DCGAN"
- "DCGAN for creative AI"
Deep Convolutional Generative Adversarial Network Examples in Context
- Artists use DCGANs to generate synthetic art pieces with unique patterns.
- Medical researchers employ DCGANs to create simulated medical images for training diagnostic systems.
- Game designers apply DCGANs to develop realistic textures and assets for virtual environments.
Deep Convolutional Generative Adversarial Network FAQ
- What is a Deep Convolutional Generative Adversarial Network?
A DCGAN is a GAN variant using convolutional layers for improved image generation. - How does a DCGAN differ from a standard GAN?
DCGANs use convolutional layers, enhancing their ability to handle image data compared to standard GANs. - What industries use DCGANs?
Industries such as gaming, art, and healthcare utilize DCGANs for content generation and image synthesis. - How do DCGANs generate images?
A generator network produces images, and a discriminator network judges their realism, iteratively refining the results. - Why are DCGANs popular in AI research?
DCGANs offer effective methods for high-quality image generation, advancing various AI applications. - Can DCGANs be used outside of image generation?
Primarily, DCGANs excel in image-related tasks, but adaptations allow usage in audio or video synthesis. - Are DCGANs difficult to train?
DCGANs require substantial computational power and tuning but are manageable with modern hardware. - What is the role of convolutional layers in DCGANs?
Convolutional layers help in processing spatial hierarchies, essential for realistic image generation. - How do DCGANs affect the creative industry?
DCGANs provide tools for artists to produce unique, AI-generated artwork, expanding creative possibilities. - Are DCGANs related to deepfakes?
Yes, DCGANs contribute foundational techniques, although deepfakes often involve more specialized GAN architectures.
Deep Convolutional Generative Adversarial Network Related Words
- Categories/Topics:
- Artificial Intelligence
- Image Generation
- Creative AI
Did you know?
In 2015, DCGANs made headlines by generating impressive synthetic images, opening doors for AI in art and media creation. This breakthrough demonstrated AI’s potential in creative fields, fostering widespread interest in generative networks.
Authors | Arjun Vishnu | @ArjunAndVishnu
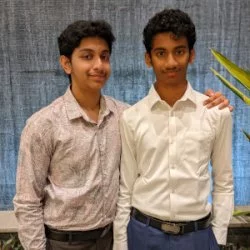
PicDictionary.com is an online dictionary in pictures. If you have questions or suggestions, please reach out to us on WhatsApp or Twitter.
I am Vishnu. I like AI, Linux, Single Board Computers, and Cloud Computing. I create the web & video content, and I also write for popular websites.
My younger brother, Arjun handles image & video editing. Together, we run a YouTube Channel that's focused on reviewing gadgets and explaining technology.
Comments (0)
Comments powered by CComment