Hard Negative Mining
Quick Navigation:
- Hard Negative Mining Definition
- Hard Negative Mining Explained Easy
- Hard Negative Mining Origin
- Hard Negative Mining Etymology
- Hard Negative Mining Usage Trends
- Hard Negative Mining Usage
- Hard Negative Mining Examples in Context
- Hard Negative Mining FAQ
- Hard Negative Mining Related Words
Hard Negative Mining Definition
Hard Negative Mining is a machine learning technique where a model is trained with "hard negatives" – examples that are close but incorrect. By focusing on these challenging cases, models improve in distinguishing subtle details between similar inputs, enhancing their performance on real-world data. This is especially valuable in applications like facial recognition and object detection, where nuanced differences are key. In practice, hard negatives are selected from a dataset or generated to challenge the model’s assumptions, improving its accuracy and robustness against similar-looking distractors.
Hard Negative Mining Explained Easy
Think of learning to spot differences in a game where two pictures look almost the same, but with small changes. Hard Negative Mining is like practicing with those tricky images to get really good at finding tiny differences. This way, AI gets better at telling things apart, even if they look almost identical.
Hard Negative Mining Origin
The concept of Hard Negative Mining emerged with advancements in computer vision and natural language processing, where fine-grained distinctions became crucial for applications. The method gained prominence as researchers sought ways to improve model accuracy by making them practice with difficult examples.
Hard Negative Mining Etymology
The term “hard negative” refers to challenging, non-matching examples that are close enough to be easily mistaken for correct ones, thereby presenting a "hard" problem for the model.
Hard Negative Mining Usage Trends
Hard Negative Mining has become more popular in recent years due to advancements in AI and the need for models that can distinguish between subtle details. From e-commerce to security applications, the technique is widely used where high accuracy is necessary for practical applications, especially in sectors relying on computer vision and text analysis.
Hard Negative Mining Usage
- Formal/Technical Tagging:
- Computer Vision
- Natural Language Processing
- Deep Learning - Typical Collocations:
- "hard negative mining technique"
- "training with hard negatives"
- "hard negative sample selection"
Hard Negative Mining Examples in Context
- In facial recognition, hard negative mining helps the model distinguish between individuals who look similar.
- Object detection algorithms improve their accuracy by training with hard negatives that are almost identical to the target object.
- In product recommendation, hard negative mining allows the system to avoid suggesting similar but irrelevant items to users.
Hard Negative Mining FAQ
- What is hard negative mining?
Hard Negative Mining is a method in AI where models train with difficult, non-matching examples to improve their accuracy. - Why is hard negative mining important?
It helps AI models become more accurate by training them with challenging examples, enhancing their ability to distinguish subtle differences. - How does hard negative mining work?
The model is presented with close-but-incorrect examples, forcing it to learn fine-grained distinctions. - Where is hard negative mining used?
Commonly in facial recognition, object detection, and recommendation systems to refine model performance. - What’s the difference between regular and hard negatives?
Regular negatives are easier to distinguish from positives, while hard negatives are more similar and challenging to identify. - Can hard negative mining be used in text analysis?
Yes, it's used to improve natural language processing by training models on difficult text examples. - Does hard negative mining make training harder?
Yes, but it also improves the model's accuracy and robustness. - Are there different methods to implement hard negative mining?
Yes, hard negatives can be selected from datasets or generated by other models. - How does hard negative mining improve model performance?
By training on difficult cases, models develop a higher level of precision. - Is hard negative mining only for supervised learning?
It's most common in supervised learning but can also be applied in other paradigms.
Hard Negative Mining Related Words
- Categories/Topics:
- Machine Learning
- Artificial Intelligence
- Model Optimization
Did you know?
Hard Negative Mining has proven essential in facial recognition, especially in settings where individuals share close physical traits, like twins. By focusing on these tough examples, AI systems can accurately distinguish between nearly identical faces, providing reliable security and identification solutions.
Authors | Arjun Vishnu | @ArjunAndVishnu
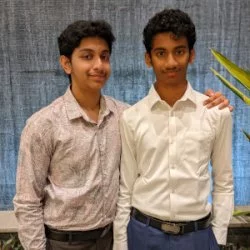
PicDictionary.com is an online dictionary in pictures. If you have questions or suggestions, please reach out to us on WhatsApp or Twitter.
I am Vishnu. I like AI, Linux, Single Board Computers, and Cloud Computing. I create the web & video content, and I also write for popular websites.
My younger brother, Arjun handles image & video editing. Together, we run a YouTube Channel that's focused on reviewing gadgets and explaining technology.
Comments (0)
Comments powered by CComment