Model Drift in Artificial Intelligence
Quick Navigation:
- Model Drift Definition
- Model Drift Explained Easy
- Model Drift Origin
- Model Drift Etymology
- Model Drift Usage Trends
- Model Drift Usage
- Model Drift Examples in Context
- Model Drift FAQ
- Model Drift Related Words
Model Drift Definition
Model Drift refers to the phenomenon where a machine learning model’s predictive performance deteriorates over time due to changes in data patterns. These changes can stem from variations in input data distribution or shifts in the relationship between input features and target outputs. There are two main types of Model Drift: data drift (input distribution change) and concept drift (output label change for the same input). Understanding and managing Model Drift is crucial to maintaining model accuracy in production environments, especially in dynamic fields such as finance, e-commerce, and healthcare.
Model Drift Explained Easy
Imagine you’re learning how to spot certain kinds of birds, but over time, the birds start looking different or new types appear. Your older examples don’t help as much anymore, so your guesses get worse. Model Drift is like that—AI systems trained on old data can start making mistakes when the world around them changes.
Model Drift Origin
The concept of Model Drift emerged as machine learning models transitioned from controlled research settings to dynamic real-world applications. Recognizing that model performance changes over time became essential as industries like finance and healthcare started using AI for critical decisions.
Model Drift Etymology
The term “Model Drift” emphasizes the gradual, often unnoticed shift in model performance over time, reflecting a model “drifting” away from its original performance.
Model Drift Usage Trends
With the widespread adoption of AI in various industries, managing Model Drift has become a key focus. Industries such as e-commerce, healthcare, and finance monitor model drift actively to ensure continued accuracy in predictions, implementing retraining or adjustment strategies to keep their AI systems relevant.
Model Drift Usage
- Formal/Technical Tagging:
- Machine Learning
- Predictive Modeling
- Model Maintenance - Typical Collocations:
- “Model drift detection”
- “Data drift management”
- “Concept drift monitoring”
Model Drift Examples in Context
- A credit risk model in banking might experience Model Drift if borrower behaviors shift due to economic changes.
- A recommender system could suffer from Model Drift as user preferences change over time, reducing the relevance of recommendations.
- Healthcare predictive models may need to address Model Drift when new health trends or treatments emerge.
Model Drift FAQ
- What is Model Drift?
Model Drift is when a machine learning model's accuracy declines due to changing data patterns over time. - How is Model Drift different from Data Drift?
Data Drift involves changes in input data distribution, while Model Drift is the broader term covering changes in model accuracy due to data or concept drift. - Why does Model Drift occur?
Model Drift happens because real-world data and relationships between features change, affecting model accuracy. - How can Model Drift be detected?
Model Drift can be detected by tracking performance metrics over time and comparing them to baseline values. - How often should models be checked for drift?
This depends on the application, but regular intervals or triggered checks after significant events are common. - Can retraining a model fix Model Drift?
Yes, retraining on new data is a common approach to handle Model Drift. - What is Concept Drift?
Concept Drift is a type of Model Drift where the relationship between inputs and outputs changes, affecting predictions. - Is Model Drift a problem in all industries?
Model Drift can impact any industry using predictive models, particularly those with rapidly changing environments like finance and retail. - What tools can help detect Model Drift?
Many machine learning platforms offer drift detection tools, and custom monitoring can be built into workflows. - How does Model Drift affect business outcomes?
Unaddressed Model Drift can lead to inaccurate predictions, affecting decisions, customer satisfaction, and potentially profits.
Model Drift Related Words
- Categories/Topics:
- Machine Learning
- Artificial Intelligence
- Predictive Maintenance
Did you know?
Model Drift was notably observed in retail AI models during the COVID-19 pandemic, where sudden shifts in customer behavior and market trends led to outdated predictions, underscoring the need for adaptive retraining and real-time model monitoring.
Authors | Arjun Vishnu | @ArjunAndVishnu
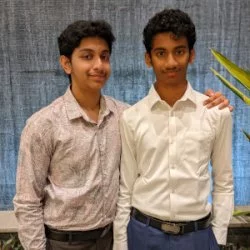
PicDictionary.com is an online dictionary in pictures. If you have questions or suggestions, please reach out to us on WhatsApp or Twitter.
I am Vishnu. I like AI, Linux, Single Board Computers, and Cloud Computing. I create the web & video content, and I also write for popular websites.
My younger brother, Arjun handles image & video editing. Together, we run a YouTube Channel that's focused on reviewing gadgets and explaining technology.
Comments (0)
Comments powered by CComment