U-Net
Quick Navigation:
- U-Net Definition
- U-Net Explained Easy
- U-Net Origin
- U-Net Etymology
- U-Net Usage Trends
- U-Net Usage
- U-Net Examples in Context
- U-Net FAQ
- U-Net Related Words
U-Net Definition
U-Net is a type of convolutional neural network primarily used for image segmentation, a task where an image is divided into meaningful parts. Designed for biomedical applications, it maps input images to desired output segments by learning contextual relationships across pixels. U-Net has an encoder-decoder structure, where the encoder captures image context and the decoder restores spatial information, helping create highly detailed segmentations.
U-Net Explained Easy
Imagine you have a coloring book with outlined pictures. U-Net is like a tool that helps to “color” these outlines based on an example image, matching colors and sections exactly. It divides and identifies parts of the picture so each section is easy to recognize.
U-Net Origin
U-Net was developed in 2015 by Olaf Ronneberger, Philipp Fischer, and Thomas Brox for biomedical image segmentation. Their goal was to create an architecture that could efficiently analyze medical images, such as identifying structures in MRI scans.
U-Net Etymology
The name “U-Net” refers to its U-shaped architecture, designed to capture both the spatial context and the details necessary for effective image segmentation.
U-Net Usage Trends
U-Net’s popularity has grown significantly due to its versatility in various industries, including medical imaging, autonomous driving, and satellite image analysis. It has become a staple in tasks requiring precise localization and segmentation, allowing AI to distinguish objects and boundaries with high accuracy.
U-Net Usage
- Formal/Technical Tagging:
- Deep Learning
- Neural Network
- Image Segmentation
- Convolutional Neural Network - Typical Collocations:
- "U-Net model"
- "segmentation with U-Net"
- "U-Net in medical imaging"
- "U-Net architecture"
U-Net Examples in Context
- U-Net is used in healthcare to identify specific tissues or structures in MRI and CT images, aiding in diagnostics.
- Autonomous vehicles use U-Net-like models to differentiate road elements, pedestrians, and obstacles in real time.
- In agriculture, U-Net helps analyze satellite images to monitor crop health and identify field boundaries.
U-Net FAQ
- What is U-Net?
U-Net is a convolutional neural network architecture developed for image segmentation tasks. - Who developed U-Net?
U-Net was developed by researchers Olaf Ronneberger, Philipp Fischer, and Thomas Brox. - Why is U-Net shaped like a “U”?
The U-shape refers to the structure that captures and restores image details through an encoder-decoder design. - Where is U-Net used?
U-Net is widely used in fields like medical imaging, autonomous vehicles, and remote sensing. - How does U-Net work?
It works by encoding image context and then decoding it to create detailed, segmented outputs. - Is U-Net suitable for real-time applications?
Yes, optimized versions of U-Net can handle real-time tasks, especially in autonomous driving. - Can U-Net segment images with high precision?
Yes, U-Net is renowned for its high precision in segmenting images due to its encoder-decoder structure. - How does U-Net differ from traditional CNNs?
U-Net’s unique encoder-decoder structure allows it to capture both spatial and contextual information. - Is U-Net only used in medical imaging?
No, U-Net is used in various fields requiring detailed image segmentation beyond medical imaging. - Can U-Net be used with 3D data?
Yes, 3D U-Net versions are available and commonly used in volumetric data analysis.
U-Net Related Words
- Categories/Topics:
- Deep Learning
- Artificial Intelligence
- Image Processing
- Convolutional Neural Networks
Did you know?
U-Net was initially created to assist in medical research, specifically in segmenting microscopic cell structures. Today, it’s vital in AI, analyzing everything from satellite images to self-driving car visuals, helping systems understand complex visual data with accuracy.
Authors | Arjun Vishnu | @ArjunAndVishnu
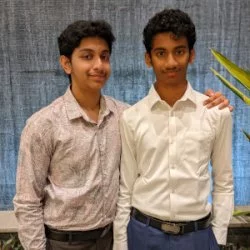
PicDictionary.com is an online dictionary in pictures. If you have questions or suggestions, please reach out to us on WhatsApp or Twitter.
I am Vishnu. I like AI, Linux, Single Board Computers, and Cloud Computing. I create the web & video content, and I also write for popular websites.
My younger brother, Arjun handles image & video editing. Together, we run a YouTube Channel that's focused on reviewing gadgets and explaining technology.
Comments (0)
Comments powered by CComment